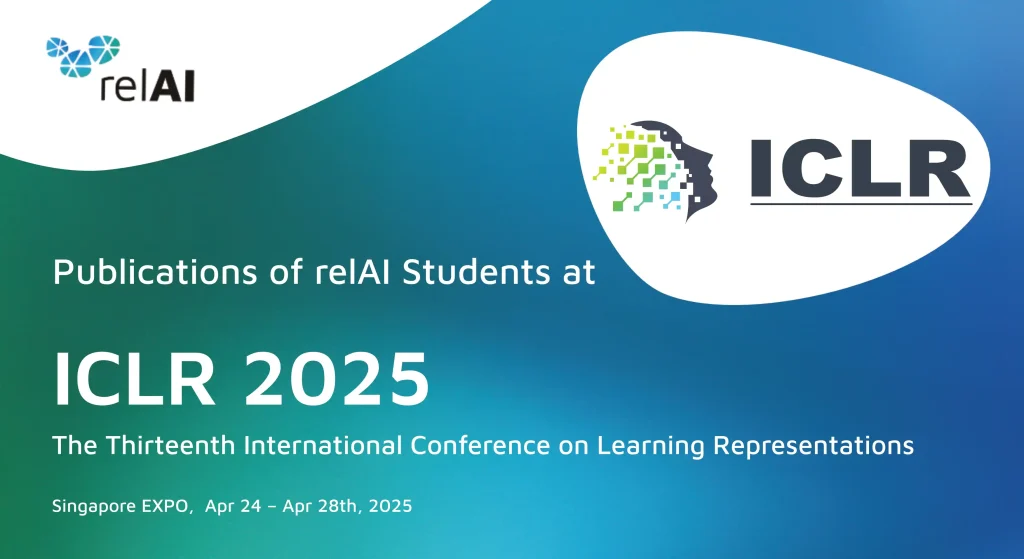
The excellent work of relAI students will be prominently represented at the Thirteenth International Conference on Learning Representations (ICLR) 2025, which will take place at the Singapore EXPO from 24 to 28 April 28 2025.
Twelve publications from our students will be presented at the conference, nine of them in the main track. Notably, four out of these nine publications have been selected for Oral or Spotlight presentations. This is a significant achievement and demonstrates the high quality of relAI research, considering that only 15% of accepted papers are invited to give a talk.
If you plan to attend the conference, do not miss the opportunity to discuss these publications directly with some of our students. Be sure to attend the Oral presentation by Yan Scholten titled "A Probabilistic Perspective on Unlearning and Alignment for Large Language Models" on the 24th April. You can learn about Lisa Wimmer´s work, "Trust Me, I Know the Way: Predictive Uncertainty in the Presence of Shortcut Learning" at the Workshop on Spurious Correlation and Shortcut Learning: Foundations and Solutions. Additionally, check out the posters of Amine Ketata and Chengzhi Hu!. Amine will be presenting his work on “Lift Your Molecules: Molecular Graph Generation in Latent Euclidean Space” and you can talk to Chengzhi Hu about “Surgical, Cheap, and Flexible: Mitigating False Refusal in Language Models via Single Vector Ablation”.
Full list of relAI publications at ICLR 2025:
- A Probabilistic Perspective on Unlearning and Alignment for Large Language Models
Yan Scholten, Stephan Günnemann, Leo Schwinn - Exact Certification of (Graph) Neural Networks Against Label Poisoning
Mahalakshmi Sabanayagam, Lukas Gosch, Stephan Günnemann, Debarghya Ghoshdastidar - Provably Reliable Conformal Prediction Sets in the Presence of Data Poisoning
Yan Scholten, Stephan Günnemann - Signature Kernel Conditional Independence Tests in Causal Discovery for Stochastic Processes
Georg Manten, Cecilia Casolo, Emilio Ferrucci, Søren Wengel Mogensen, Cristopher Salvi, Niki Kilbertus - Differentially private learners for heterogeneous treatment effects
Maresa Schröder, Valentyn Melnychuk, Stefan Feuerriegel - Surgical, Cheap, and Flexible: Mitigating False Refusal in Language Models via Single Vector Ablation
Xinpeng Wang, Chengzhi Hu, Paul Röttger, Barbara Plank - ParFam -- (Neural Guided) Symbolic Regression via Continuous Global Optimization
Philipp Scholl, Katharina Bieker, Hillary Hauger, Gitta Kutyniok - Lift Your Molecules: Molecular Graph Generation in Latent Euclidean Space
Mohamed Amine Ketata, Nicholas Gao, Johanna Sommer, Tom Wollschläger, Stephan Günnemann - Constructing Confidence Intervals for Average Treatment Effects from Multiple Datasets
Yuxin Wang, Maresa Schröder, Dennis Frauen, Jonas Schweisthal, Konstantin Hess, Stefan Feuerriegel - Trust Me, I Know the Way: Predictive Uncertainty in the Presence of Shortcut Learning
Lisa Wimmer, Bernd Bischl, Ludwig Bothmann
Workshop on Spurious Correlation and Shortcut Learning: Foundations and Solutions - Privacy Amplification by Structured Subsampling for Deep Differentially Private Time Series Forecasting
Jan Schuchardt, Mina Dalirrooyfard, Jed Guzelkabaagac, Anderson Schneider, Yuriy Nevmyvaka, Stephan Günnemann
Workshop on Advances in Financial AI: Opportunities, Innovations and Responsible AI - Cracking the Code: Evaluating Zero-Shot Prompting Methods for Providing Programming Feedback
Niklas Ippisch, Anna-Carolina Haensch, Markus Herklotz, Jan Simson, Jacob Beck, Malte Schierholz
Workshop on Human-AI Coevolution - Exact Certification of (Graph) Neural Networks Against Label Poisoning
Mahalakshmi Sabanayagam, Lukas Gosch, Stephan Günnemann, Debarghya Ghoshdastidar
VerifAI: AI Verification in the Wild
Oral Presentation - Main Track
Spotlight Presentation - Main Track
Posters - Main Track
Workshops